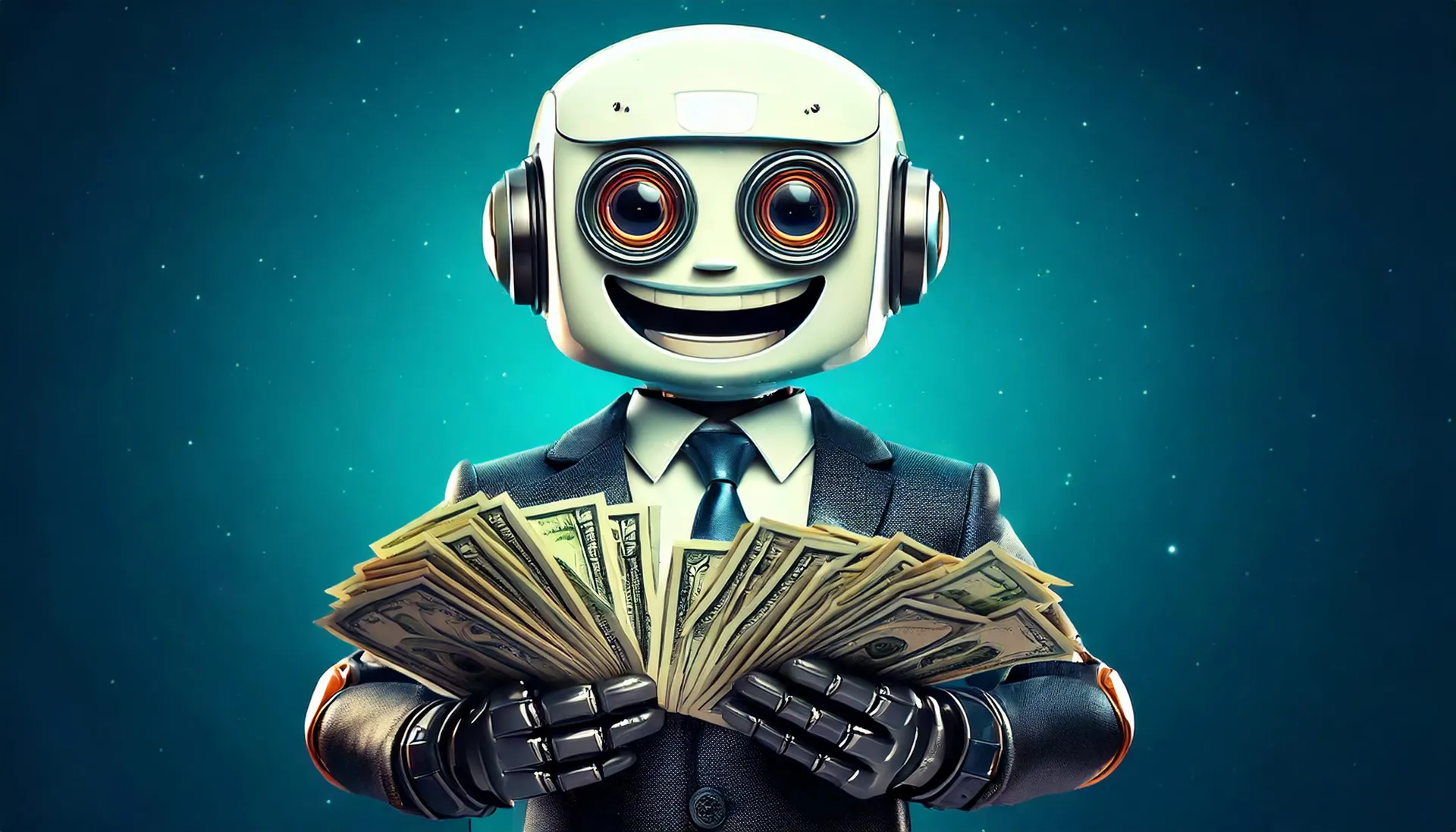
Identifying High-Value AI Use Cases
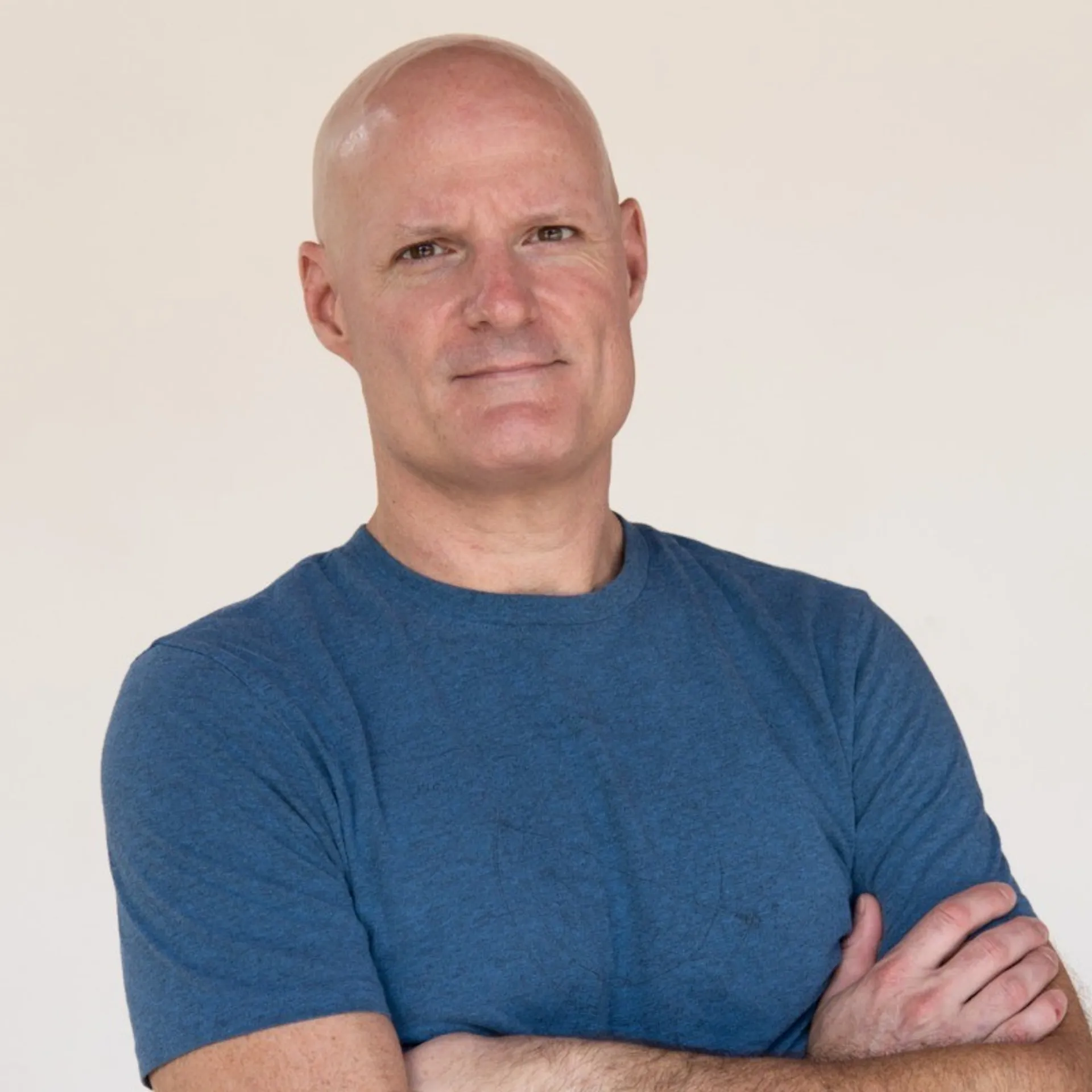
Jayson Ambrose
Founder & CEO, Big Robot
According to RSM US, reality is setting in for artificial intelligence after months of hype. Companies developing AI tools face critical questions: What does your AI actually do? What return can I expect on my investment? How does your gadget differentiate you from the other guys?
Understanding the Current Landscape
The latest McKinsey Global Survey on AI says that 65% of organizations now regularly use AI, nearly double from ten months ago. While most of the respondents predict AI will lead to significant industry changes, the path to value isn't obvious. These companies report both cost decreases and revenue increases, but McKinsey notes that only a small subset of of them attribute more than 10% of their earnings to AI projects.
Business Reporter emphasizes that despite significant progress in capabilities, AI is not a magic bullet. Many AI projects fail to move beyond the tinkering stage, and those that do often require substantial resources to implement effectively. The current IT landscape is already crowded with apps, and many businesses are struggling with data quality and old-school systems.
This tension between potential and reality reflects what Matthew Ball, CEO of Epyllion, describes in RSM US: pinpointing the onset of a new technological era can be tricky. Tech integrates into daily life in small ways that aren't immediately noticeable. Changes build momentum gradually until it becomes obvious only in hindsight that a new era has emerged and you're living in it. We don't want to get caught with our pants down.
Ok, you've got the big picture. Now how do you find the right opportunities?
Framework for Identifying AI Opportunities
The success of AI adoption depends on identifying the right opportunities. While Wavestone emphasizes starting with strategic alignment, our approach combines principles from Lean methodology and Jobs-to-be-done to ensure projects address real user needs and business challenges.
1. Start with Jobs to be Done
Begin by identifying the core jobs your customers and employees need to accomplish:
- Map current process steps and workflows
- Document pain points and inefficiencies
- Measure time spent and error rates
- Define desired outcomes clearly
2. Apply Lean Problem Analysis
Use the "5 Whys" technique to understand each identified problem deeply:
- Why is this a problem worth solving?
- Why does it occur repeatedly?
- Why haven't current solutions worked?
- Why would AI be more effective?
- Why is now the right time to address it?
3. Evaluate AI Potential
For each identified job or problem, assess three key areas:
Data Readiness
- Availability of relevant historical data
- Quality and structure of existing data
- Capability to collect needed data going forward
Problem Characteristics
- Presence of clear pattern recognition elements
- Frequency of repetitive decision-making
- Potential value from automation
Implementation Feasibility
- Required technical capabilities
- Integration with existing systems
- Risk factors and mitigation strategies
4. Prioritize High-Impact Opportunities
Review potential projects through multiple lenses:
- Strategic alignment with business goals
- Potential for measurable impact
- Resource requirements and availability
- Implementation complexity
- Time to value
Focus on selecting 2-3 opportunities that offer the best combination of impact and feasibility for initial projects.
High-Impact AI Use Cases
You came for the use cases. Here are the goods:
1. Customer Experience and Service
The Financial Times reports how Octopus Energy transformed their customer service using AI:
- Automatic transcription and response generation for customer queries
- 50% of customer service emails now AI-assisted
- Improved response times and account health metrics
Gartner highlights two additional successful implementations:
Healthcare: Mayo Clinic
- AI-enabled medical chatbot conducts dynamic patient interviews
- Efficiently gathers symptom information and medical history
- Provides personalized care recommendations
- Reduces clinician burnout through streamlined intake
Banking: Ally
- Contact center assistant
- Automates post-call note-taking and summarization
- Reduces manual documentation work
- Enables associates to focus on customer interactions
- Improves speed and accuracy of call documentation
2. Business Operations and Strategy
The Times highlights how Grind, a medium-sized coffee retailer, integrates AI across their operations:
- Marketing content generation
- Customer query management
- Performance report creation
- Company-wide AI training program for staff
3. Process Automation and Efficiency
The Guardian highlights how Employment Hero uses AI to transform HR processes:
- Automate new hire onboarding and contract organization
- Complete payroll system setup in 20 seconds
- Handle CV screening for both volume and complexity
- Enable HR staff to focus on team integration
Survey results show:
52% report increased efficiency 42% see faster operations 65% reduce paper usage
4. Documentation and Knowledge Management
Deloitte identifies several high-impact applications for medium-sized businesses:
- Legal document automation
- Technical documentation generation
- Policy and procedure updates
- Knowledge base maintenance and updates
Each of these applications shares common success factors:
- Clear alignment with business objectives
- Measurable impact on efficiency or revenue
- Focus on augmenting rather than replacing human capabilities
- Structured approach to implementation and training
Common Challenges and Solutions
McKinsey's research identifies several key challenges:
-
Accuracy Concerns
- 44% of organizations have experienced negative consequences from AI use, with inaccuracy being the most common issue
-
Risk Management
- Only 18% of organizations have an enterprise-wide council for AI governance decisions
- Just one-third require AI risk awareness for technical talent
-
Implementation Time
- Most organizations need one to four months to put AI into production
- Time varies by business function and level of customization
Next Steps for Medium-Sized Businesses
The experiences of companies like Grind and Octopus Energy show that successful AI implementation requires four key elements:
- Start with real problems, not technology solutions
- Use data you already have rather than creating new data streams
- Begin with 2-3 focused projects where success is clearly measurable
- Give teams time to learn and adapt to new ways of working
By focusing on specific business problems and measuring results carefully, we can move past the hype to find practical applications that deliver real value.
The most successful organizations treat AI as a tool to enhance human capabilities, not replace them. For deeper insights into AI implementation strategies and updates, follow the referenced sources throughout this article.